Statistical Modeling
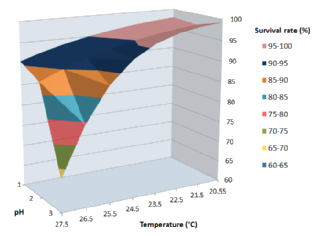
Multiple Logistic Regression Modeling
The UCD Aquatic Health Program (AHP) offers a novel epidemiological approach to analyzing environmental studies using multiple logistic regression. This statistical approach allows researchers to estimate survival rates or other outcomes that include different independent predictor variables. One substantial benefit to using this method is improved precision and accuracy by minimizing bias from known confounders. This method has been used previously to simultaneously assess many potential predictors which were recorded during a long term research project from 2006-2008. The final model reflects significant effects of water quality parameters such as dissolved oxygen, electric conductivity, temperature and their interactions on Hyalella azteca survival.
Currently AHP researchers and statisticians are investigating aquatic contaminant interactions in binary mixtures using multiple logistic regression. These studies along with continued work regarding more complicated contaminant mixtures effects in an environmentally relevant scale will be used to protect California’s delicate ecosystem.
This epidemiological approach can be implemented in a large variety of studies with improved outcomes such as:
- Predicting survival rates among different species by including water quality parameters,
- Diminishing electrical conductivity effect as a confounding factor,
- Analyzing interaction effects among binary and tertiary contaminant mixtures,
- Including infinite predictive variables in bioassessment studies.
The UCD AHP has the statistical expertise and computational resources to perform multivariate analysis of experimental results and exploratory multivariate analysis of aquatic environmental data. These, as well as new and innovative experimental designs, can be used on an extremely large scale. The AHP is practiced in performing standardized USEPA aquatic toxicity statistical protocols, yet also takes pride in creating comprehensive analyses customized to the needs of each client and data set.